AI and Machine Learning for ANSYS Simulations Webinar Series Part 1: Modern Design of Experiments
Thu, Jun 5, 2025 1:00 PM - 2:00 PM CDT
Getting the most out of ANSYS simulations requires efficient use of the available simulation budget as well as validating that the simulation produces results that agree with reality. This webinar series will discuss the role design of experiments, surrogate modeling, and machine learning (ML) tools such as statistical calibration can play in calibrating ANSYS simulations to physical data, validating their accuracy, and maximizing the knowledge gained from their use.
With surrogate modeling, first an ML model is trained to predict the results of a particular ANSYS simulation. This ML model is then used in place of the ANSYS simulation to run any desired analyses. The ML model’s rapid prediction of the ANSYS simulation results allows more analyses to be performed and in less time.
In this first webinar of 4, SmartUQ’s modern, efficient design of experiment (DOE) tools for deciding which data to collect for ML model training will be discussed. Topics covered will include DOEs for batch collection of data, to facilitate multi-fidelity modeling approaches, for problems with input constraints, and for simulation model calibration. SmartUQ’s Adaptive DOEs which utilize an existing ML model to intelligently decide where further data should be collected in order to have the largest effect on improving the ML model’s accuracy will also be discussed.
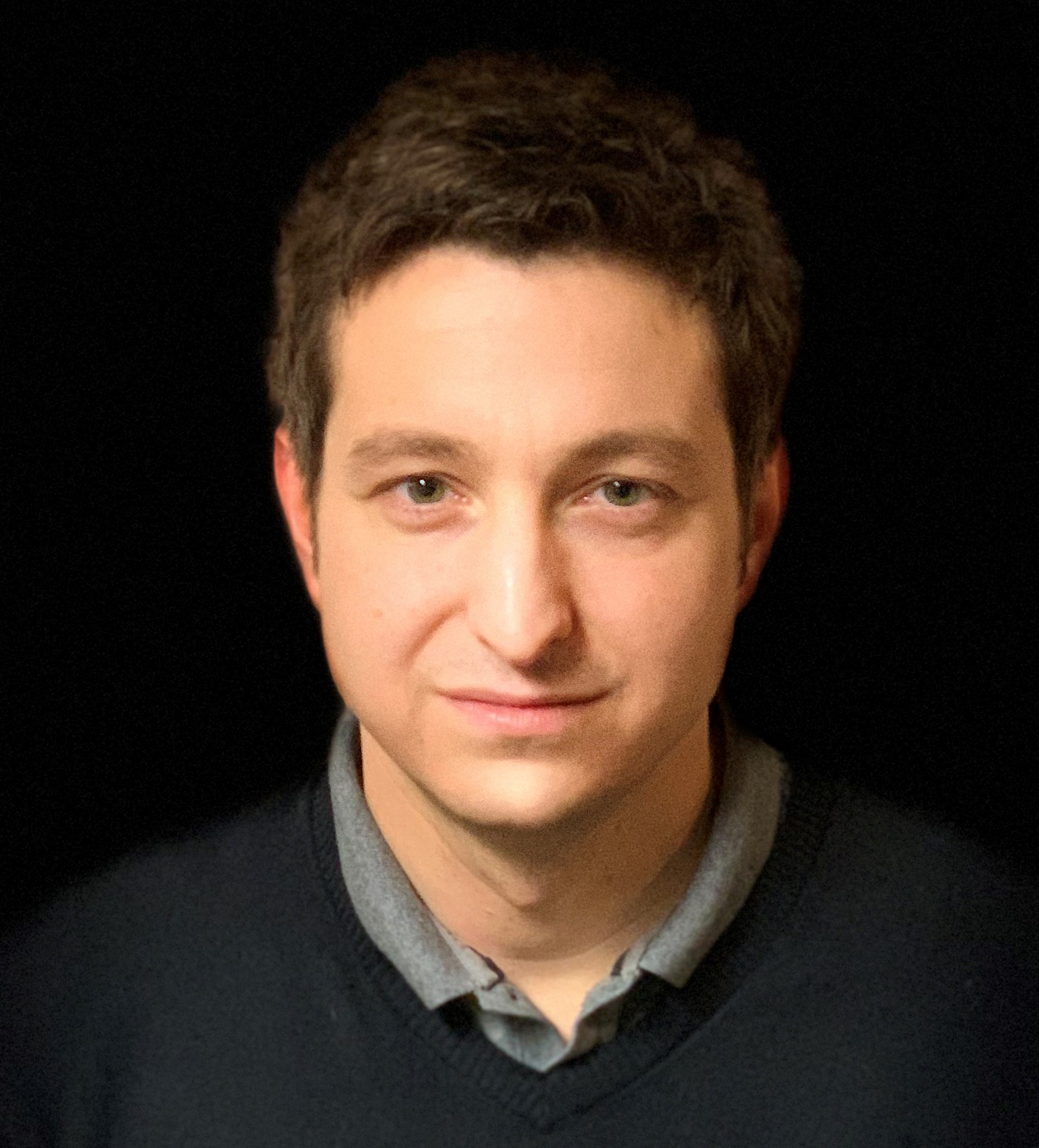
Presented by Gavin Jones, Principal Application Engineer
Gavin Jones serves as a Principal Application Engineer at SmartUQ, where he is responsible for performing simulation and AI work for clients in the automotive, aerospace, defense, semiconductor, and other industries. He is a member of the SAE Chassis Committee as well as the AIAA Digital Engineering Integration Committee. Gavin is also a key contributor in SmartUQ’s Digital Twin/Digital Thread initiative.